Data modernization POC provides improved and immediate access to predictive insights, built with an out-of-the-box solution
Solution highlights
Client: USDA FPAC
Need: USDA FPAC’s workforce lacks access to data tools, has limited training to analyze and extract insights from data, and is limited in data science resources.
Solution: Cadmus built a custom data pipeline, which aggregates, processes, and applies ML models to data from multiple disparate FPAC and geological data sources, in a few weeks using out-of-the-box vSTART components.
Impact
- Data access, analytics, and management improved
- Data quality issues resolved
- Advanced analytics made consumable by intuitive BI dashboards for multiple use cases
- Data is now consumed through the pipeline at scale
- End-users, data engineers, data scientists experienced improved workflows and efficiency in setting up and communicating results, creating a proactive data analytics culture
Key data sources used in the FPAC POC
- Snow Telemetry (SNOTEL), Snow Course Data & Products
- Soil Climate Analysis Network (SCAN) Data & Products
- CropScape – Cropland Data Layer
- Modified Common Land Unit (CLU)
- Cropland Imagery
- National Agriculture Imagery Program (NAIP)
- Gridded National Soil Survey Geographics (gNATSGO) Database
- Complete coverage of the best available soil information: 70 GB of soil data converted to TIFF format
Key aspects of the technical architecture for the FPAC POC
- Kubernetes cluster to serve as an orchestrator for storage and services accounts
- Implemented Infrastructure as Code (IaC) – Terraform scripts to automate the creation of storage accounts
- Python code to ingest data through SOAP API and stream the data using Azure event hub to simulate Telemetry data
- Azure Databricks as a Delta Lake Solution
- Azure Databricks Notebooks used to define data quality and aggregation jobs
- Azure Databricks storage is used to store both hot & cold data
- Visualization and spatial data modeling is implemented using Power BI, ArcGIS Pro, and JupyterLab
- Machine Learning is implemented using MLFlow to provide predictive analytics over a wide range of data such as crop delineation.
The challenge
Many federal agencies struggle with a cumbersome legacy data infrastructure, excessively dispersed data, limited scientific tooling, and a reactive culture toward data analytics. In addition to these challenges, USDA FPAC’s workforce is limited in data science resources, lacks access to data tools, and has little training to analyze and extract insights from data.
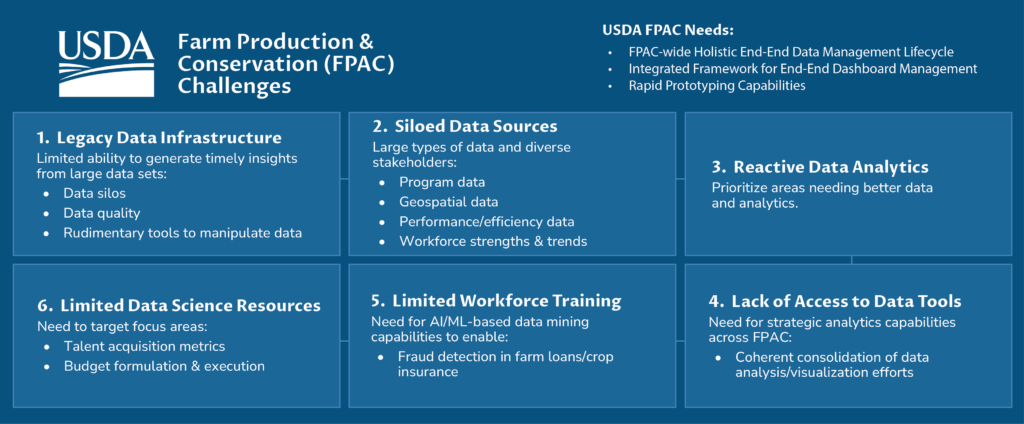
To address these challenges, the agency is on a mission to transform and modernize its end-to-end data management platform, culture, processes, and tools. FPAC possesses large amounts of valuable real-time and historical data and has embarked on a journey to harness and maximize the power of this data in the service of a diverse set of stakeholders.
The solution
As a proof of concept (POC) for FPAC, Cadmus leveraged vSTART, an internal platform of out-of-the-box components, to build a custom data pipeline in a tight timeline. This POC consolidates disparate data sources, consumes batch and streaming data, uses Delta Lake layers to improve quality and aggregation challenges, and streamlines the ability to create dashboards and visualizations of varying complexity for multiple use cases. By leveraging vSTART, Cadmus was able to quickly and efficiently build a robust data pipeline that can handle large volumes of data and provide valuable insights for various business needs.
“The POC approach followed a consistent Cadmus strategy of applying Agile and UCD principles throughout the product lifecycle. This approach ensured that we focused on the customer experience while satisfying business objectives. By leveraging reusable components from vSTART, we designed and built the minimum viable product (MVP) within weeks,” said Khanh Armstrong, Cadmus Director of Corporate IP.

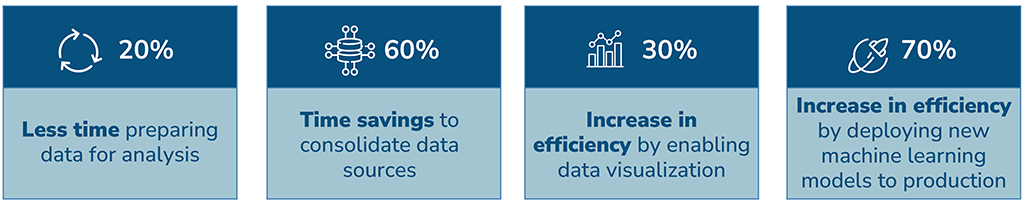
Cadmus’ overarching technical strategy and the architecture for this POC reflect our understanding of FPAC’s vision of a data-driven digital transformation mission. The POC itself provides easy and immediate access to a powerful combination and overlays of weather, crop, soil, NAIP imagery data from 2015 through 2019 available at their fingertips via data visualization tools with data exporting and sharing capabilities.
We consider this POC to be a minimum viable product for a much larger data pipeline solution that can be incrementally built to cater to FPAC’s custom needs. The technical architecture for this POC provides foundational technical components while retaining the flexibility to develop additional functionality.
“Cadmus’ architecture leverages a best-in-class technology stack, bringing all data to one platform with the ability to perform data governance and lay the foundation for developing advanced, powerful analytical and visualizations tools on top of assured quality of underlying data,” said Sarma Musty, Cadmus Data Architect.
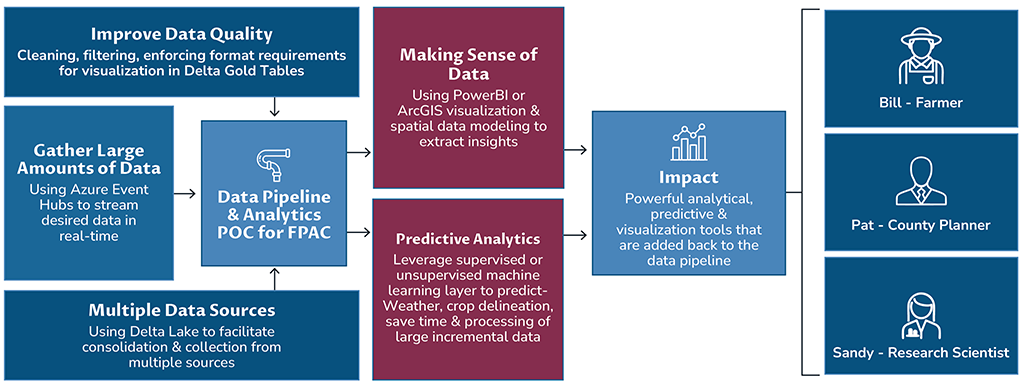